Sharpening Your Edge: An Intro to Advanced Sports Betting Analytics
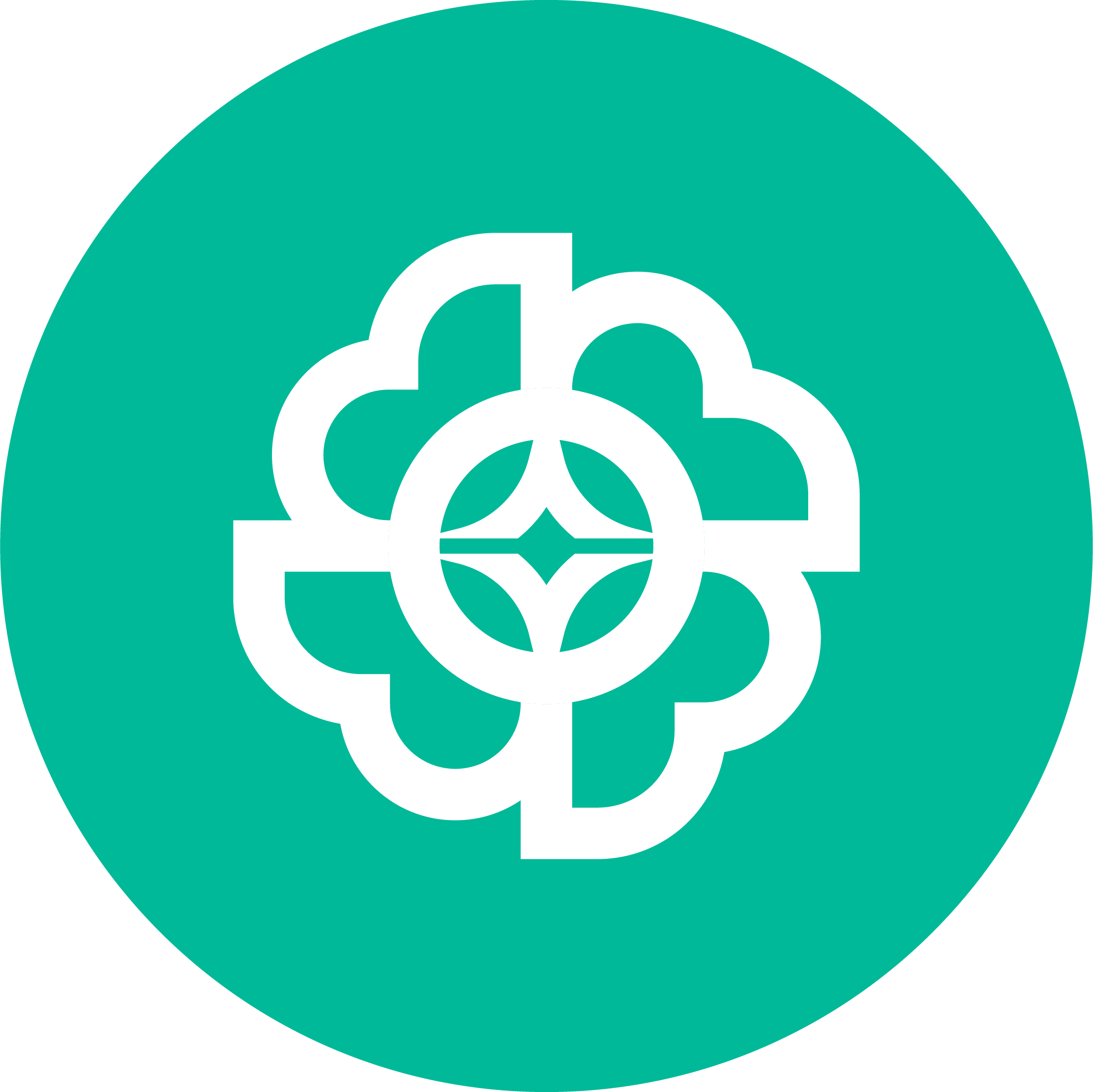

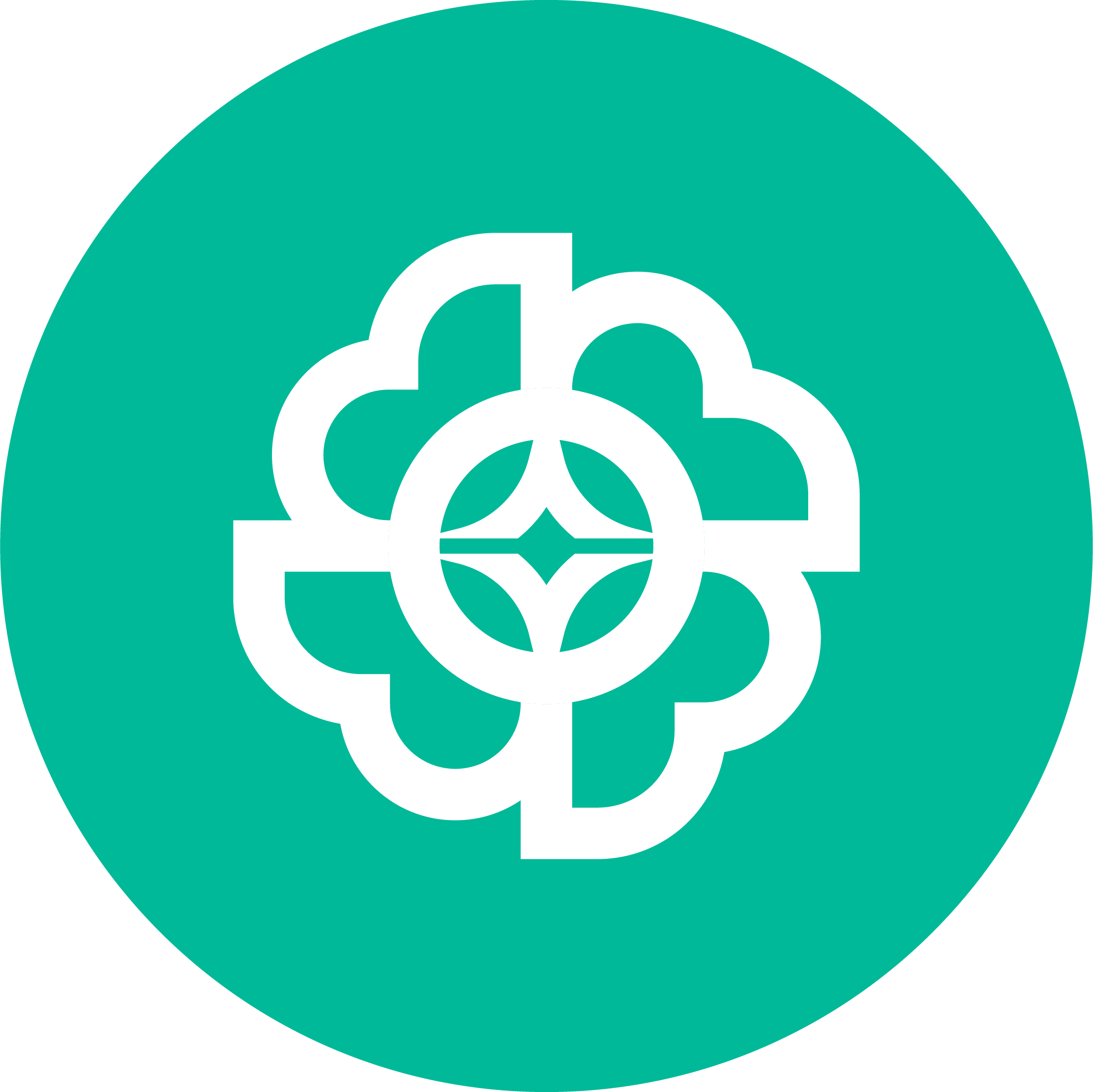
The Rise of the Quantitative Bettor
The world of sports betting is in the midst of a quantitative revolution. Gone are the days of handicapping games with gut instinct and traditional wisdom alone. Today's sharpest bettors are leveraging advanced mathematical models and big data to identify mispriced lines and inefficiencies in the betting market.
In this introduction, we'll explore some of the key concepts and techniques that underpin this new wave of analytical sports betting. Whether you're a seasoned handicapper looking to up your game or a sports fan intrigued by the intersection of data and betting strategy, grasping these principles is essential to competing in the modern betting landscape.
Core Concepts in Sports Betting Analytics
+EV Decision Making
The most foundational concept in quantitative sports betting is positive expected value (+EV). In simplest terms, a +EV bet is one where the probability of winning multiplied by the amount you stand to win is greater than the probability of losing multiplied by the amount you stand to lose.
At its core, successful betting is about consistently making +EV decisions. A bettor who only places +EV bets, sized appropriately for their edge and bankroll, is virtually guaranteed to profit in the long run, even if many of those bets lose. Analytically-minded bettors strive to build models that accurately estimate true event probabilities, then compare those probabilities to available betting odds to identify +EV opportunities.
Efficiency Metrics
Traditionally, sports bettors and fans have focused on basic statistics like points scored, batting average, or yards gained. But these raw counting stats can be misleading, as they don't account for the pace of play or the strength of the opposing team or consider the game situation.
Advanced bettors prefer efficiency metrics that measure a team's or player's per-possession or per-play effectiveness, adjusted for the opposition faced. Examples include points per possession in basketball, yards per play in football, and wRC+ (weighted runs created plus) in baseball. By focusing on efficiency over raw production, bettors can get a truer sense of a team's underlying performance level that is more predictive of future results.
Pythagoras in Cleats
One particularly useful team-level efficiency metric is Pythagorean expectation. Originally developed for baseball but now applied to other sports, this formula estimates a team's expected winning percentage based on the points they've scored and allowed.
The insight behind Pythagorean expectation is that a team's point differential is often a better predictor of future performance than their actual win-loss record, which can be skewed by lucky wins in close games. Many bettors compare a team's actual record to their Pythagorean expectation to identify teams that have over- or under-performed relative to their underlying statistics and may be due for positive or negative regression.
Cluster Luck
Another important concept in advanced sports betting is the role of luck and random variance. Over a small sample of games, a team or player's results can be heavily influenced by factors outside their control, like the bounce of an oddly shaped ball or a referee's blown call.
Sharp bettors look to quantify this luck factor to identify teams whose recent results may not be sustainable. One common approach is to look at a team's record in close games, typically defined as games decided by one score or less. Since the outcome of close games is often determined by just a few key plays, teams that win a high percentage of close games are often benefiting from good fortune that is unlikely to continue. Conversely, teams that have lost a lot of nail-biters may be better than their record indicates and poised for positive regression.
Putting Analytics into Practice
Building an analytical sports betting model is a complex undertaking, but the core steps are:
-
Gather relevant data: Collect and clean granular data on team and player performance, with an emphasis on efficiency metrics and adjusted for strength of schedule.
-
Identify predictive factors: Use statistical techniques to determine which metrics are most correlated with future performance and betting outcomes.
-
Construct a predictive model: Build a mathematical model that weights the predictive factors to estimate each team's true performance level and forecast game outcomes.
-
Convert performance estimates into probabilities: Use historical data on how often teams with certain characteristics win to map performance estimates to win probabilities.
-
Compare probabilities to betting odds: Calculate the implied probability of the available betting odds and look for discrepancies between your model's estimates and the market prices.
-
Allocate bets based on edge size: Bet more when your model shows a large edge, and less (or not at all) when the edge is small or nonexistent.
In practice, this process is never as straightforward as it sounds. Sports are dynamic and unpredictable, and even the most sophisticated models are working with imperfect information. But by taking a rigorously analytical approach, sports bettors can gain a meaningful edge on the traditional wisdom of the crowd.
Challenges and Limitations
While the rise of analytics has undoubtedly transformed sports betting, it's important to recognize some key challenges and limitations of a purely quantitative approach:
-
Data quality: The output of any statistical model is only as good as its input data. Issues like small sample sizes, changes in playing style or personnel, and the inconsistent availability of advanced data can limit a model's predictive power.
-
Non-stationary environments: Sports leagues are constantly evolving, with rule changes, strategic innovations, and shifting trends in play style. A model trained on historical data may struggle to adapt to these changes.
-
Opponent adjustments: The best coaches and teams are skilled at making tactical adjustments to exploit their opponent's weaknesses. These adjustments can be difficult to capture in a model based on season-long statistics.
-
Efficient market hypothesis: As analytical techniques become more widespread, their insights should theoretically be incorporated into the betting lines, making it harder to find mispriced bets. There's an ever-present risk of looking for an edge that has already been arbitraged away by other sharp bettors.
Despite these challenges, the broad shift towards analytical sports betting shows no signs of slowing. And for bettors willing to embrace the complexities and uncertainties that come with a quantitative approach, the potential rewards are significant. In a multi-billion dollar industry where the majority of bettors lose over the long run, a sustainable edge is extremely valuable. By combining an analytical mindset with deep domain knowledge and a disciplined approach to bankroll management, the modern sports bettor can build that elusive edge and turn a profit in an increasingly competitive market.